‘Humans in the loop’: RLHF and how real people are quietly training generative AI
As generative AI continues its seepage into just about everything, Oscar Quine (who freelances for both The Drum and AI companies) looks into the hidden human element in honing its output.
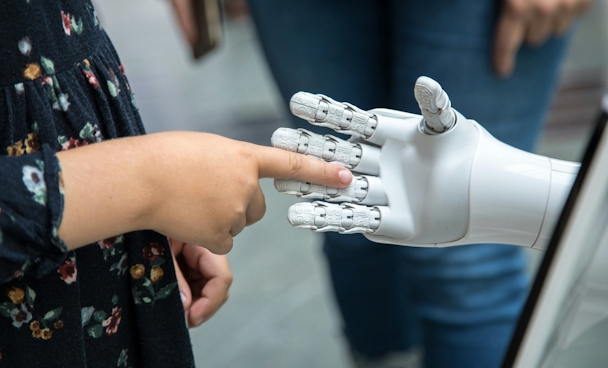
Thousands of real people around the world are quietly 'training' the next generation of conversational AI / Katja Anokhina via Unsplash
When OpenAI first devised ChatGPT, success was by no means assured. The pursuit of artificial intelligence (AI) had been underway for decades. The Silicon Valley unicorn (at the time a non-profit with early commitments from the likes of Elon Musk and LinkedIn’s Reid Hoffman) had arrived on a model that seemed to work on a small scale. Proceeding to pump more data into it, increasing the parameters underlying its outputs, the result seemed to work: the company had created a coherent, knowledgeable (or ‘knoweldgeable’) chatbot.
Many question marks still exist around the inner workings of large-language models (LLMs), even within programmer communities – the model arrived on by OpenAI is something of a black box, as are its competitors. And at this stage of their development, there’s an element of chance or randomness to the model’s output. Hallucinations, mistakes, and other inaccuracies occur, inevitably reducing trust in the products.
In the race for generative and conversational AI that doesn’t make these kinds of errors, the stakes (and investments) are sky high. OpenAI was valued last month at $157bn, and it’s estimated the company spends $3bn a year training ChatGPT. To mitigate error, much of this spend goes on human input: real, flesh-made people, ‘training’ the AI to do better.
Want to go deeper? Ask The Drum
RLHF: The human factor in AI training
Alongside my freelance work for The Drum, I spent parts of the last year working for agencies that facilitate this human training for some of the most advanced and best known LLMs. The scale of the human input in these operations is astonishing: hundreds of writers and editors crafting tens of thousands of bespoke conversations to be fed into the AI to improve its final output. Seeing these teams felt a little bit like going behind the curtain in the Wizard of Oz.
So, how much can be done to shape the responses an AI gives? And with brands an publishers alike increasingly incorporating chatbots into their brand experience, what can be done to ensure AIs stay on-script, safely representing those businesses?
The work I was involved in was part of what’s known as reinforced learning from human feedback (RLHF). One aspect of this involves training a reward model by rating the responses given by LLMs against one other, or on a numeric scale. The second element is supervised fine-tuning, whereby example conversations are written and inputted into the LLM.
All this human training, says Bob Briski, global senior vice president of AI at Dept, can be an expensive endeavor. “That data is very expensive. It's maybe $10 or $15 per multi-turn conversation, which, if you need at least 5,000 that gets to be $50,000-100,000 pretty quickly. And that’s just the minimum for that kind of work... But, as you can guess, it's a minority of customers that are at the point where they’re actually fine-tuning.”
(These costs naturally mean that players in the AI space are figuring out how AI might be able to take on the task of training, too. Just a few weeks ago, Meta announced that it has discovered a holy grail: the long sought-after mechanism by which AIs can improve themselves, without the need for this human input.)
Briski estimates there are only around 10 companies building ‘foundational’ LLMs being from scratch. While the large-scale work I was involved in myself is relatively rarefied, for companies repurposing LLMs for more focused purposes – a legal assistant or triage nurse, say – a degree of fine-tuning might still be needed. The question then becomes which model to use: GPT4.0, for example, has greater general knowledge than 3.5, but that earlier, nimbler model can prove easier to fine-tune.
For many, says James Wolman, head of data science at Braidr, fine-tuning is usually the ‘icing on the cake’. He explains: “It’s the last stage you get to once you’ve prototyped and got a working model. It takes a long effort to get to that stage.”
Advertisement
RAG and prompt-tuning
Companies looking to hone LLMs for set tasks, or to communicate on behalf of their brand, can use other methods such as retrieval-augmented generation (RAG) and prompt-tuning.
RAG, says Braidr’s Wolman, is “the poster child of gen AI at the moment”. The process involves giving an LLM a special dataset to draw from, focusing its output around a certain pool of knowledge. Braidr, he says, recently built an ‘AI-driven, next-generation search intelligence’ for healthcare brand Haleon, which involved Wolman and his colleagues building just such a dataset.
“It would learn from that,” Wolman explains. “So if we could see that people had previously tagged-up toothpaste using ‘whitening group’, it would know that that’s something it can carry forward.”
Prompt-tuning is another example of relatively low-intensity input, whereby a model is pre-loaded with ‘soft prompts’ which inform how it responds to later inputs. This allows for a model to be personalized, without the need for specialized changes to its underlying architecture.
Advertisement
Conversational design and brand safety
When it comes to brand safety, Joe Crawforth, head of research and development at Jaywing, says it’s important to always maintain a ‘human in the loop’. “Let us help you build those guardrails around it to make sure it's safe,” he says. “Never take it at face value to say you’ve created a content machine and just have it rolling out content constantly without any kind of human review.”
In a recent roundtable for The Drum Network, Andy Eva-Dale, chief technology officer at Tangent, discussed the rise of conversational design. He described it as a medium concerned with “how you convey a brand through conversation or tone of voice.” He says this is more important than ever – and that it was pushing creatives into new territory.
Increasingly, the question may not be what a branded chatbot says to you, but how it says it. Empathic Voice Models (EVMs) promise to pick up on emotions in a user’s voice and respond with a suitably emotional response. Last month, OpenAI launched Advanced Voice Mode, promising: “more natural, real-time conversations that pick up on non-verbal cues, such as the speed you’re talking, and can respond with emotion”.
To this end, James Calvert, head of generative AI at M&C Saatchi, reveals his agency is working on a voice model for a fast-food brand that will be able to take orders, allowing drive-throughs to stay open 24/7. Of course, the voice will have to say the right things, in precisely the right way.
Calvert stresses the project is still very much in the developmental stage. While turning out fast food might be well-automated, the quest for a voice assistant to help customers through the sales process is in its infancy.
“No one’s got this right yet,” he says.
Content created with:

DEPT®
DEPT® is a pioneering technology and marketing services company that creates integrated end-to-end digital experiences for brands such as Google, KFC, Philips,...

Tangent
From shaping the underlying strategy to refining the final design and build, we create experiences that enhance people’s lives, prioritise sustainable digital...

Jaywing
At Jaywing, we’ve made it our mission to help clients establish concrete foundations in a world of shifting sands. As a data-powered integrated agency, we bring...